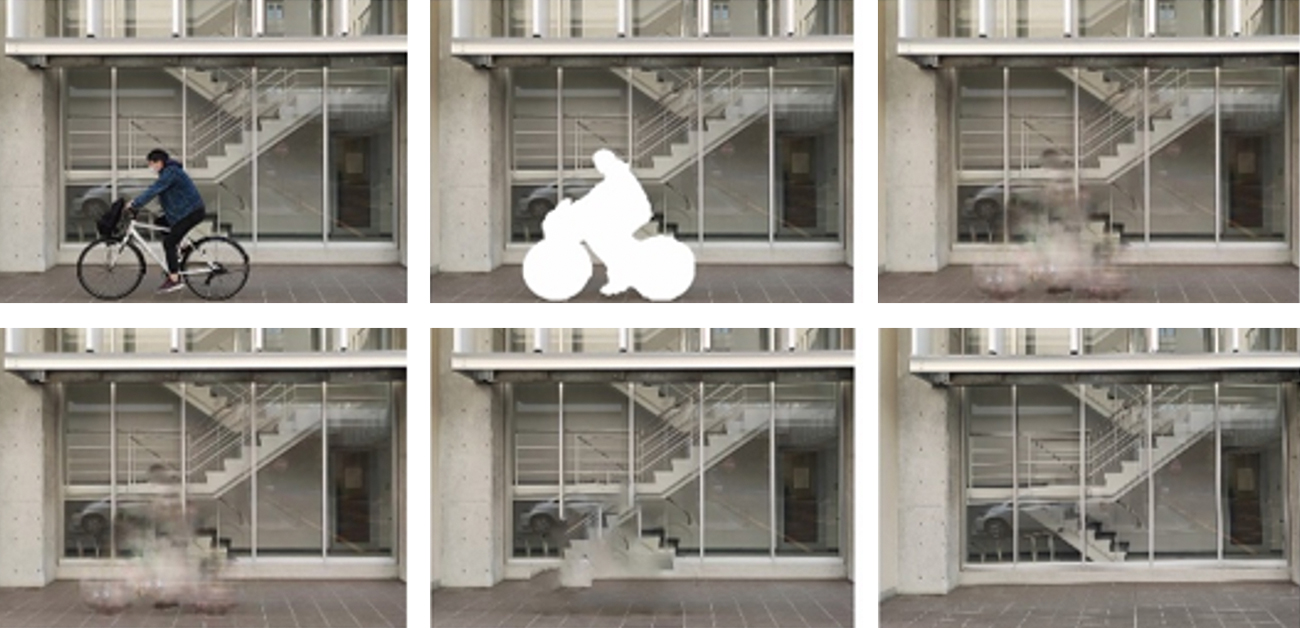
X-ray street vision
Researchers at Osaka University create a custom dataset of building facades to train a machine learning algorithm to digitally remove unwanted objects, which may lead to advancements in automatic image reconstruction technology
Scientists from the Division of Sustainable Energy and Environmental Engineering at Osaka University used generative adversarial networks trained on a custom dataset to virtually remove obstructions from building façade images. This work may assist in civic planning as well as computer vision applications.
The ability to digitally “erase” unwanted occluding objects from a cityscape is highly useful but requires a great deal of computing power. Previous methods used standard image datasets to train machine learning algorithms. Now, a team of researchers at Osaka University have built a custom dataset as part of a general framework for the automatic removal of unwanted objects — such as pedestrians, riders, vegetation, or cars — from an image of a building’s façade. The removed region was replaced using digital inpainting to efficiently restore a complete view.
The researchers used data from the Kansai region of Japan in an open-source street view service, as opposed to the conventional building image sets often used in machine learning for urban landscapes. Then they constructed a dataset to train an adversarial generative network (GAN) for inpainting the occluded regions with high accuracy. “For the task of façade inpainting in street-level scenes, we adopted an end-to-end deep learning-based image inpainting model by training with our customized datasets,” first author Jiaxin Zhang explains.
The team used semantic segmentation to detect several types of obstructing objects, including pedestrians, vegetation, and cars, as well as using GANs for filling the detected regions with background textures and patching information from street-level imagery. They also proposed a workflow to automatically filter unblocked building façades from street view images and customized the dataset to contain both original and masked images to train additional machine learning algorithms.
This visualization technology offers a communication tool for experts and non-experts, which can help develop a consensus on future urban environmental designs. “Our system was shown to be more efficient compared with previously employed methods when dealing with urban landscape projects for which background information was not available in advance,” senior author Tomohiro Fukuda explains. In the future, this approach may be used to help design augmented reality systems that can automatically remove existing buildings and instead show proposed renovations.
Fig.1 Results of automatic object removal and facade completion. (a) People, (b) rider, (c) vegetation, (d) car. (credit: © 2021 Jiaxin ZHANG et al., IEEE Access)
Fig. 2 Workflow for automatic object removal and obstructed facade completion using semantic segmentation and generative adversarial inpainting. (credit: © 2021 Jiaxin ZHANG et al., IEEE Access)
Fig. 3 Collection method for a perpendicular street facade. (a) Road networks, (b) sampled points from the centerline of the road, (c) deflection angle θ, and (d) orthographic facade obtained from a street view service. (credit: © 2021 Jiaxin ZHANG et al., IEEE Access)
The article, “Automatic object removal with obstructed façades completion using semantic segmentation and generative adversarial inpainting” was published in IEEE Access at DOI: https://doi.org/10.1109/ACCESS.2021.3106124.
Related Links
FUKUDA Tomohiro (Researchers Database)
EurekAlert!
AlphaGalileo