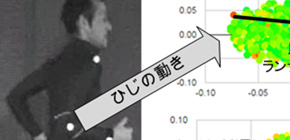
Basic technology for passing down traditional skills through computers developed
Supporting acquisition of motor skills through use of big data of motion and artificial intelligence techniques
A group of researchers from the University of Tsukuba, Osaka University, and Mizuno Corporation (Osaka) developed technology for instructing the path to ideal movement in order to improve sports techniques based on "Big Data of Motion," a collection of data obtained using sensors and films of motion. (Patent pending)
Graduate School of Systems and Information Engineering, University of Tsukuba -- YAMAGIWA Shinichi , Associate Professor
The Institute of Scientific and Industrial Research, Osaka University -- KAWAHARA Yoshinobu , Associate Professor
This group of researchers discovered that there were differences in the movements of the elbows, knees, and ankles between experienced marathon runners and beginners by expressing running movement data of some 2,000 runners owned by Mizuno as numerical skill values by employing artificial intelligence technology.
Based on these findings, this group developed a technology called “skill grouping” for supporting acquisition of skills by displaying the effects of movements in an easily-understood scoring format.
Furthermore, this group found that by applying skill grouping to baseball batting motions, the difference in tools could be expressed as numerical values. In other words, the difference in the effect of these tools on movement has come to be expressed in numerical values.
In addition, when applying skill grouping to the data of ski parallel turns measured by a motion sensor, even in games in which the scoring system was based on appearance alone, differences in athletic ability have become able to be quantified. It was also found that differences in numerical values in several trials by experienced athletes were small, and that it was possible to quantify that they always performed the same motions.
Applying skill grouping to daily exercise will enable time-sequential management of healthcare and athletic ability control. Previously, it was difficult to produce general-purpose health information devices because there were no objective standards on being healthy; however, skill grouping has enabled such devices to be developed. These devices will become tools necessary for health management in the era of the Internet of Things.
Furthermore, if skill grouping is applied to inheriting traditional performance arts and design skills, the inheritance of technical tradition, which is a worldwide urgent challenge, may be realized through a new system using artificial intelligence.
Abstract
Capturing human movement has become available in detail due to the advancement of motion sensor technology integrated by micro-machine and also due to the one of optical recording by high speed and high resolution image sensors. Therefore, we can easily record the human activity as the body movement BigData and analyze it to quest skill to become an expert of a target body movement. Especially, in the sports activity, the quest for becoming an expert athlete has been tried by using a mathematical model of an ideal body movement experienced from the biomechanics approach. The skill is discussed by comparing the differences from the predicted coordinates of body parts captured during the target performance. However, the approach potentially includes difficulties such as modeling the body control from the dynamics system for all human movements. And also the approach needs for adjusting jitters of the individual characteristics. Therefore, when applying the conventional approach, we must discuss a huge number of combinations of mathematical models and then we would find a model for the ideal body movement. To overcome the difficulty, this paper proposes an approach to visualize skill differences among experts and beginners from the BigData called the skill grouping method. It exploits the skill groups clustered by machine learning approach based on a kernel method. This paper shows applications of the skill grouping method from sports activities. Those show validities for finding the skill differences comparing to the BigData of skillful athletes, and also the one for managing skill transition of an athlete in a timeline.
This image shows a running scene. (Photo courtesy of University of Tsukuba)
Thesis title: " Skill Grouping Method: Mining and Clustering Skill Differences from Body Movement BigData "
Publication: Proceeding of International conference on BigData 2015, IEEE. (October 29-November 1, 2015 • Santa Clara, CA, USA)
Related link