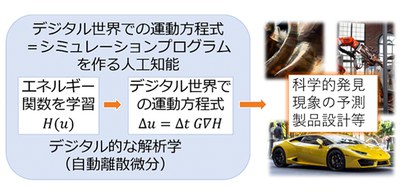
Simulations faithful to physical laws run by AI can replicate conservation and dissipation of energy using digital analysis
A group of researchers of Kobe University and Osaka University developed a simulation technology to make a model faithful to physical laws from observation data of phenomena whose mechanism and equation are unknown, using artificial intelligence.
Commonly, predictions of physical phenomena are carried out via simulations using supercomputers, and these simulations are run using algorithms and equations based on the laws of physics. These equations are versatile but cannot fully replicate characteristics of individual phenomena.
If observation data of phenomena are used for simulations using artificial intelligence (AI) technology, it will become possible to run simulations for each phenomenon and improve the accuracy of simulation predictions. However, in traditional prediction technology using AI, computers were able to understand only digital data, so it was difficult to apply physics of the real world to computers in order to fully replicate phenomena while obeying physical laws such as energy conservation laws.
In this study, the researchers developed a new AI technology that allows for predictions of phenomena while obeying the energy conservation laws. This method incorporated physical laws that can hold in the digital world.
The group newly developed the digital version of automatic differentiation backpropagation*, which is used for machine learning, thereby replicating physical laws such as energy conservation laws in the digital world.
*Backpropagation algorithm: A way to compute the gradients needed to fit the parameters of a neural network. Backpropagation is a special case of an extraordinarily powerful programming abstraction** called automatic differentiation (AD).
**Abstraction in mathematics is the process of extracting the underlying structures, patterns or properties of a mathematical concept or object.
The method this group developed enables adherance to the energy conservation laws in simulations by AI. Using this technology will make it possible to produce highly reliable predictions. In this technology, AI learns energy functions from observation data of physical phenomena and creates equations of motion in the digital world. These equations of motion can be used as-is in simulation programs, realizing R&D using the programs.
In addition, it is not necessary to rewrite the equation of motion for computer simulations, so physical laws such as energy conservation laws can also be replicated. The researchers took geometric approaches such as symplectic geometry or Riemannian geometry in order to put the laws of physics into the computer, thereby significantly expanding the range of phenomena to be predicted.
The researchers expanded the usage of this method so that they could also handle energy dissipation phenomena. Thus, it has become possible to estimate the rate of energy decrease at high accuracy. It is anticipated that AI technology to predict such phenomena will be realized. The researchers increased efficiency of AI’s learning rate; experimentally increased the learning speed 10 times faster than that of conventional methods.
This group’s achievements were presented at the Thirty-fourth Conference on Neural Information Processing Systems (NeurIPS 2020), which was held from December 7, 2020. 9454 papers were posted to NeurIPS2020. Of the top 1900 theses selected, this paper was one of 105 oral presentations in the top 1.1%.
Figure 1
Figure 2
The article, "Deep Energy-based Modeling of Discrete-Time Physics," will soon be published in Advances in Neural Information Processing Systems 33 (NeurIPS 2020).