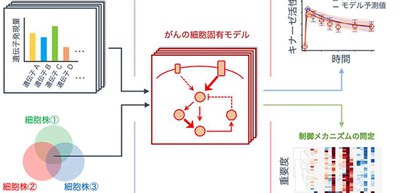
BioMASS, a framework for modeling, simulation, and parameter estimation will accelerate personalized medicine
A group of researchers from Osaka University’s Institute for Protein Research developed a calculation method for creating patient-specific models to realize personalized medicine. With this method, they developed BioMASS (Modeling and Analysis of Signaling Systems), a computational platform for prediction and analysis of signaling dynamics using RNA-sequencing (RNA-seq) gene expression data.
Traditional cancer treatment was chosen based on tumor size, degree of invasion, and biomarker; however, since tumor classification has a high impact on patient outcome, new tumor classification based on gene expression data of individual patients is imperative.
Thus, this group of researchers aimed at cancer classification, namely classifying genome data according to gene characters and therapeutic response and building patient-specific models for personalized medicine.
Based on the hypothesis that gene expression levels are tightly linked with and quantitatively control the dynamics of signaling networks regardless of the cell type, they developed a computational method to predict the signaling dynamics from RNA sequencing (RNA-seq) gene expression data.
By using the RNA sequences of cancer cell lines obtained from the Cancer Cell Line Encyclopedia (CCLE) database and cellular simulations, this group developed a computational method to quantitatively predict the signaling dynamics of kinase, which is an enzyme that phosphorylates proteins and the most important drug target.
This method allows for the prediction of signaling activities of untrained cell lines through training of experimental data of multiple breast cancer cell lines. Thus, the model that learned the parameters from other cells can accurately predict the dynamics of the untrained cells using only the gene expression data, increasing the efficiency of drug screening.
If more experimental data is added to the BioMASS framework and mathematical models and analysis methods are sophisticated and expanded, it will become possible to build a patient-specific model for cancer classification simply by entering autologous RNA-seq data into mathematical models trained using cell lines, thereby making it possible to find the most suitable medications for the patient.
The application of these mathematical models for in silico drug screening techniques helps in the identification of disease mechanisms and quantitative analysis. This technique will play a complementary role in drug discovery using artificial intelligence (AI), which expertly works with data analytics in categorizing data. BioMASS can be downloaded for free from here.
Based on BioMASS, the researchers constructed an in silico patient-specific model (virtual patient) that combines mathematical modeling and patient clinical data. BioMASS will allow for the construction of mathematical models and simulation analysis and from the simulation results, subtype classification, prognosis classification, and drug susceptibility analysis will become possible.
The development of this study will create a great breakthrough in the acceleration and cost reduction of drug development as well as personalized medicine.
Figure 1
FIgure 2
The article, " A computational framework for prediction and analysis of cancer signaling dynamics from RNA sequencing data—Application to the ErbB Receptor Signaling Pathway," has been published in the Cancers (Basel) at DOI: 10.3390/cancers12102878.