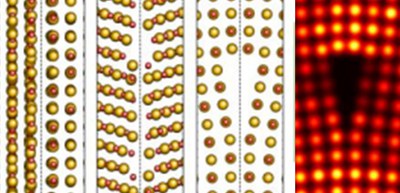
Accurate prediction of thermal conductivities of grain boundaries from local atomic environments using machine learning techniques
A group of researchers from the Japan Fine Ceramics Center (JFCC), the National Institute for Materials Science (NIMS), Osaka University, and Nagoya University has constructed a model for accurately predicting thermal conductivities of grain boundaries (GBs) directly from local atomic environments (LAEs) using computational science and machine learning (ML) techniques.
Nearly all metals and ceramics are polycrystalline materials that consist of many crystals (the grains). Since a GB is a structural defect and GBs separate regions of different crystalline orientation within a polycrystalline solid, GBs affect the electrical and thermal conductivity of the material as a whole.
Using advanced microscopy coupled with novel computer simulations, this group investigated atomic structure near GBs and thermal conductivities for more than 90 types of MgO GBs.
In this study, the researchers demonstrated that a microscopic structure metric, the local distortion factor (LDF), correlated well with atomically decomposed thermal conductivities obtained from perturbed molecular dynamics for a wide variety of MgO GBs.
Based on this correlation, using ML techniques, such as structure descriptors capable of distinguishing different GB structures and hierarchical clustering to identify LAEs, they constructed a model for accurately predicting thermal conductivities of GBs.
They classified the structural environments of atoms at the cores of different GBs into groups suitable for constructing their ML model, finding that classifying the LAEs into six groups was sufficient for accurate prediction of GB thermal conductivities. They demonstrated that GB thermal conductivity could be predicted with high precision from their local atomic structures alone.
Their model is not just a prediction model. It clarified the local distortion factor (LDF) in thermal conductivities as well. A microscopic structure metric (or LDF) correlated well with atomically decomposed thermal conductivities. The model revealed that small distortions to local atomic environments were sufficient to reduce overall thermal conductivity dramatically.
This group’s model will enable more precise nanoscale designing of thermally functional materials. Their achievements will optimize the production process of thermoelectric conversion materials and thermal barrier coating materials and improve highly integrated electronic devices.
Figure 1
The article, “Quantitative prediction of grain boundary thermal conductivities from local atomic environments,” was published in Nature Communications at DOI: http://doi.org/10.1038/s41467-020-15619-9.