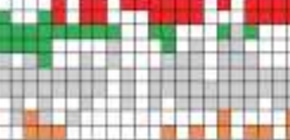
A good night’s sleep
Osaka University researchers use smartphones and machine learning to measure sleep patterns
Despite spending at least one quarter to one third a day sleeping, good sleep can elude many people, and the diagnosis and treatment of sleep disorders remains primitive. Osaka University researchers have designed new technology that uses machine learning to model a personal sleep pattern based on the sounds made during sleep. Because the sounds can be recorded at home with no fancy devices, it is expected that doctors using this technology could diagnose patients under normal sleeping conditions, which is expected to lead to better treatment.
Patients with sleep disorders are often evaluated by polysomnography (PSG), which measures an assortment of the body’s activity during sleep, including brain activity, eye movement, and heart rhythms. Osaka University Associate Professor Ken-ichi Fukui calls PSGs ineffective because they take the patient out of his natural sleeping environment.
“Our environment influences how we sleep. We should not expect the same patterns sleeping at a hospital or sleeping at home.”
Fukui and other researchers at the Institute of Scientific and Industrial Research, Osaka University, collaborated with researchers at the Dental School. The sounds of sleeping dental students were recorded using smartphones placed bedside. Fukui then used a novel machine learning algorithm prepared by his group to analyze the sounds and compare them with PSG data taken from the same sleeping students.
“We modified self-organizing map (SOM) algorithms to visualize the dynamics of sleep,” he said.
The SOMs extracted very obvious sleeping patterns, as subjects showed clear changes in their sounds with their sleep stage. Snoring was evident during deep sleep periods, whereas some of the sleepers tended to grind their teeth during light sleep periods.
“Visualization by SOM makes it very easy to detect abnormal patterns in sleeping,” said Fukui.
Associating sleep sounds with sleep patterns provides a whole new prospect of sleep diagnostics.
“There are lots of devices that assess sleep,” Fukui notes, “but none are scientifically proven, and they usually require you wear the device,” which he adds discourages their use.
Fukui expects that apps for smartphones and tablets can be used to record sleep sounds and convert the information into recommended adjustments to the home, such as lighting or room temperature, for better sleep.
Abstract
The quality of a good sleep is important for a healthy life. Recently, several sleep analysis products have emerged on the market; however, many of them require additional hardware or there is a lack of scientific evidence regarding their clinical efficacy. We proposed a novel method via clustering of sound events for discovering the sleep pattern. This method extended conventional self-organizing map algorithm by kernelized and sequence-based technologies, obtained a fine-grained map that depicts the distribution and changes of sleep-related events. We introduced widely applied features in sound processing and popular kernel functions to our method, evaluated their performance, and made a comparison. Our method requires few additional hardware, and by visualizing the transition of cluster dynamics, the correlation between sleep-related sound events and sleep stages was revealed.
Figure 1. Comparison between sleep pattern visualization by AI method (above) and sleep stage by PSG (below), showing correlation between sleep-related sounds and sleep stages.
To learn more about this research, please view the full research report entitled “ Personal Sleep Pattern Visualization via Clustering on Sound Data ” at this page of the AAAI 2017 Workshop Proceedings website.
Related link