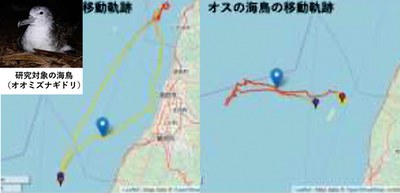
DeepHL, a deep learning-assisted platform for the comparative analysis of animal movement data developed
A group of researchers from Osaka University, Nagoya University, Doshisha University, Nagoya City University, Hokkaido University, Okayama University, and Tokyo University of Agriculture developed DeepHL, a web-based open system to support the comparative analysis of big behavioral data acquired by or for biologists.
The researchers tested the platform on a variety of trajectories of roundworms (Caenorhabditis elegans), insects (red flour beetles, Tribolium castaneum), mice, bears, and seabirds (streaked shearwaters), revealing new movement features of these animals.
Recent advances in sensing technologies, such as the Global Positioning System (GPS) and computer vision, provide “big behavioral data” of animals; however, there was a risk of overlooking important features because big behavioral data required substantial effort for biologists to analyze manually and depended on their experience and intuition.
In this study, the researchers leveraged the feature learning capacity of deep learning, or learning of the high-level feature extraction processes performed within a deep neural network (DNN), which was originally conducted by experienced researchers.
Figure 1 shows example outputs of DeepHL when the researchers compared trajectories from male seabirds to those from female seabirds. DeepHL automatically finds trajectory segments characteristic of each sex, highlighting some characteristic segments of the trajectories in red.
In examining the red flour beetle, Tribolium castaneum (T.castaneum), which is known for tonic immobility (TI, or “death-feigning” to an antipredator), the researchers established the strains with short (S strain) and long (L strain) duration of TI in T.castaneum. The test results indicated the difference in strategies for survival between the S- and L-strain beetles. The L-strain beetles can survive because of their long duration of TI against predators, while the S-strain beetles attempt to escape from a predator by frequently changing their moving directions. Thus, the researchers assumed that S-strain beetles selected a survival strategy of changing movement directions to escape from predators.
In comparing a trajectory of a normal mouse and a trajectory of a 6-hydroxydopamine (OHDA) lesion mouse model of Parkinson’s disease (PD), characteristic behavior of normal mice that visited places far away from the initial position of the trajectory was detected by the DNN. This suggests that PD mice do not prefer exploring unvisited locations, although normal mice and rats spontaneously prefer to explore an environment, particularly in novel places. DeepHL revealed that the abnormal behavior of PD mice hinders such spontaneous behavioral traits.
Comparative methods are the most fundamental approaches to animal behavior analysis and biologists use these methods for various analysis of the influence of diseases, gene deficiency, and weather on behavior. The use of this software to quantify animal behavior will facilitate the understanding of diseases common to humans and animals and a better understanding of human-wildlife conflicts due to climate change will help prevent or alleviate conflicts.
Figure 1
Figure 2
The article, “Deep Learning-assisted Comparative Analysis of Animal Trajectories with DeepHL,” was published in Nature Communications at DOI: https://www.nature.com/articles/s41467-020-19105-0#MOESM6.
Related links
Hara Laboratory, Department of Multimedia Engineering, Graduate School of Information Science and Technology, Osaka University (link in Japanese)