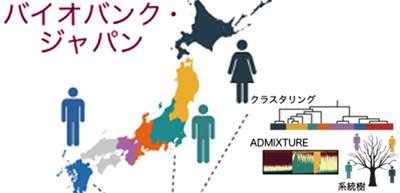
Regional diversity in Japanese population clarified using machine learning for large-scale genomic data
Clarification of genome will pave the way for the development of personalized medicine
A group of researchers from Osaka University and RIKEN has unveiled methods to visualize subtle genetic differences within a population by applying machine learning algorithms to genomic genotypes from Japan, the United Kingdom (UK), Malaysia, and some Arab States.
Specifically, by applying dimensionality reduction methods for data visualization (i.e. machine learning algorithms: PCA, t-SNE, PCA-t-SNE, UMAP, and PCA-UMAP) to biobank-derived genomic data of populations, the researchers reduced data of individuals on the two-dimensional space.
PCA -- principal component analysis
t-SNE -- t-distributed stochastic neighbor
UMAP -- uniform manifold approximation and projection
They also demonstrated the possibility that genomic diversity might have an impact on polygenic risk score (PRS), a method to estimate the risk of a disease or disease-related trait for an individual.
Anatomically modern humans migrated out of Africa to Europe, the Middle East, and Asia. The history of migration, admixture, and adaptation shapes the diversity recorded in the human genome. Thus, it is possible to roughly identify races of people in the world through principal component analysis (PCA), a classical linear dimensionality reduction method, of genetic data. However, to what degree our genomes differ due to subtle environmental and regional diversity was not well known.
This research group proposed methods to visualize regional diversity on a two-dimensional space by applying recently developed machine learning methods to genome data of 170,000 people collected from various regions throughout Japan.
Using these methods, they discovered that the Japanese population was roughly separated into two groups: Hondo (primarily in the mainland) and Ryukyu (Okinawa and part of Kyushu). The application of PCA–UMAP to the Ryukyu cluster dissolved it into different sub-clusters.
The researchers also applied these five dimensionality reduction methods to genotype data from the UK, Malaysia, and some Arab States, identifying subpopulations within each population.
In addition, they demonstrated that the fine-scale population structure in the Japanese population also affected the PRS, suggesting that a careful assessment of the genetic and phenotypic architecture of complex traits was necessary when planning the risk stratification of individuals based on the relative rank of PRSs.
This study showed that the PRS differences might reflect biases from the uncorrected structure and that it was also necessary to understand the adaptive evolution in regions and their history. This will give a critical suggestion to tailor-made medical treatment in the future.
Figure 1
Figure 2
Figure 3
The article, "Dimensionality reduction reveals fine-scale structure in the Japanese population with consequences for polygenic risk prediction," was published in Nature Communications at DOI: https://www.nature.com/articles/s41467-020-15194-z.
Related Links
Department of Statistical Genetics, Graduated School of Medicine, Osaka University (link in Japanese)