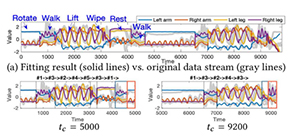
Streaming algorithm realizing smart factory via quick data analysis and future forecasting
A group of researchers from Osaka University developed StreamScope, a world-class innovative streaming algorithm, by advancing their time-series big data analysis technique.
Data mining using this algorithm in production will offer: predictive analytics, data driven process optimization, real-time machine learning, information extraction, data analysis, and result illustration. These had not been realized by conventional deep-learning-based techniques.
As shown in connected cars (vehicles designed to be connected to the Internet), digital twins (a virtual representation of a real-world object), and materials informatics (an emerging cross-disciplinary field aimed at combining materials science and data science) in the development of devices and materials, industry and society have drastically changed. In such a setting, AI (artificial intelligence) and big data analysis are anticipated as techniques to support the 4th industrial revolution. In the fields of manufacturing, automobile, finance, medical care, and retailing, software for processing images and video, natural language, and sensor data, is actively developed using deep learning technique, a subfield of AI. However, because there are many technological challenges in deep learning, its scope of application has been limited.
This group developed a technique for performing data analysis and predictive analytics at high speeds and with a high degree of accuracy by identifying patterns in data streams as events happen and by modelling a series of events. This technique allows one to monitor important dynamic factors and predict future outcomes through real-time identification of major patterns and latent trends (regimes) and extraction of a dynamic relationship between regimes from big time-series data, including sensor data streams in Internet of Things (IoT).
Their predictive analytics using machine learning for data modelling achieved the world’s best forecasting accuracy and computational time among existing advanced predictive analytics and deep learning. For example, for manufacturing data, this streaming algorithm can compute 670,000 times faster and 10 times more accurately (forecasting error reduced by 88%), compared to the latest predictive analytics using deep learning.
By further developing and utilizing this technique, it will become possible to perform real-time data analysis for quick and automatic detection of early signs of accidents and other problems, such as sudden braking and steering actions while driving and equipment breakdown at smart factories. Currently, this group is engaging in joint research with several companies on smart factories, vehicle driving data analysis, and biological information analysis.
Their research results were presented at the largest international data mining workshops: ACM SIGKDD2019 (on August 6, 2019) and ACM CIKM2019 (on November 5, 2019).
Figure 1
Figure 2
Figure 3
Related links
- Dynamic Modeling and Forecasting of Time-evolving Data Streams Dynamic Modeling and Forecasting of Time-evolving Data Streams Dynamic Modeling and Forecasting of Time-evolving Data Streams Dynamic Modeling and Forecasting of Time-evolving Data Streams Dynamic Modeling and Forecasting of Time-evolving Data Streams Dynamic Modeling and Forecasting of Time-evolving Data Streams Dynamic Modeling and Forecasting of Time-evolving Data Streams Dynamic Modeling and Forecasting of Time-evolving Data Streams Dynamic Modeling and Forecasting of Time-evolving Data Streams Dynamic Modeling and Forecasting of Time-evolving Data Streams
- Automatic Sequential Pattern Mining in Data Streams
- Sakurai & Matsubara Laboratory, The Institute of Scientific and Industrial Research, Osaka University (link in Japanese)