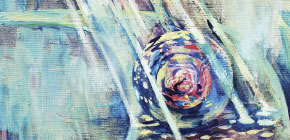
Machine learning-based high-speed fluorescence imaging cell sorter developed with Ghost Motion Imaging technology
A group of researchers from The University of Tokyo, Osaka University, and ThinkCyte, Inc. developed an ultrahigh-speed fluorescence imaging–activated cell sorter. They created the sorter by combining a single-pixel imaging method based on compressive sensing (a technique to reconstruct signals representing cell shape data by sinc interpolation at a high speed and resolution) with machine learning and computational fluid dynamics technology, separating morphologically similar cell types at ultrahigh-speed (more than a thousand times faster than a conventional microscope).
A technique for analyzing and selectively separating a large amount of morphologically similar cell types is increasingly sought after. However, manual separation of cells using a microscope has limited practical use. In flow cytometry technology employed in cell sorting, a cell sorter can measure the amount of fluorescence emitted by each cell, but it cannot measure and analyze fluorescence images of cell morphology. Thus, a cell sorter capable of measuring and analyzing morphological information obtained by fluorescence imaging techniques has been sought after.
With the new concept of “capturing the image of an object using motion of the object on structured illuminations,” the researchers developed the Ghost Motion Imaging method, a single-pixel imaging method based on compressive sensing, which permits imaging of even dark objects, such as fluorescence, at a high speed (more than 10,000 images per second).
Under the assumption that if image analysis is done by computers automatically without human intervention, data in a format that humans can understand is not necessary, the researchers developed a simple, accurate, and high-speed real-time imaging data processing method to allow a machine model to directly classify single-pixel compression signals to reconstruct images using machine learning.
This Ghost Motion Imaging technique enables (1) measurement of high-speed fluorescence cell imaging, (2) real-time analysis by machine learning, and (3) selective isolation of cells in microfluid. This technique allows for high-precision classification and separation of cells and high-speed detection and isolation of cancer cells from other cells in the model blood.
At the start of the development of this technique, the researchers founded a venture, ThinkCyte, Inc., beyond the borders of academic fields and organizations. Through joint research with the company, the researchers completed a machine learning-based fluorescence “imaging”–activated cell sorting (FiCS) in which hardware and software are integrated. Clinical research for this system will begin this academic year and ThinkCyte, Inc. will start to offer its prototype for research from the next academic year.
Morphological evaluation, selection, and usage of a large number of cells with this technique will contribute to medical practice such as making diagnoses using blood/body fluids, regenerative medicine, and cell therapy in which high levels of safety and reliability are required.
This study is a pioneering example of efforts toward the international commercialization of a product. In addition, it is notable that the researchers themselves attempt to create industries and markets in which specialists such as Ph.D. holders can thrive. This project will serve as a good example of nationwide industry-academia-government collaboration.
Abstract
Ghost imaging is a technique used to produce an object’s image without using a spatially resolving detector. Here we develop a technique we term “ghost cytometry,” an image-free ultrafast fluorescence “imaging” cytometry based on a single-pixel detector. Spatial information obtained from the motion of cells relative to a static randomly patterned optical structure is compressively converted into signals that arrive sequentially at a single-pixel detector. Combinatorial use of the temporal waveform with the intensity distribution of the random pattern allows us to computationally reconstruct cell morphology. More importantly, we show that applying machine-learning methods directly on the compressed waveforms without image reconstruction enables efficient image-free morphology-based cytometry. Despite a compact and inexpensive instrumentation, image-free ghost cytometry achieves accurate and high-throughput cell classification and selective sorting on the basis of cell morphology without a specific biomarker, both of which have been challenging to accomplish using conventional flow cytometers.
Cell in Ghost Cytometry © 2018 Sacco Fujishima, Creative Commonslicense CC BY-ND
Figure 1
To learn more about this research, please view the full research report entitled " Ghost cytometry " a t this page of Science .
Related links